Enhanced Wind Forecasting
Overview
The massive penetration of weather-dependent power generation from renewable energy sources (RES) creates several challenges for power system operation. The short-term forecasting of RES generation, a few minutes up to days ahead, is a cornerstone prerequisite for the secure and economic operation of power systems with high RES-penetration.
In the past two decades, considerable research has been conducted leading to several forecasting tools, most of them originating from the specific field of a particular RES. Wind power forecasting techniques were developed through several R&D projects (FP5 Anemos, FP6 Anemos.plus, FP7 SafeWind, SMART4RES) funded by the European Commission, whereas solar forecasting is being addressed through the FP7 DNICast and FP7 Performance Plus projects. Spinoffs have emerged providing forecasting services, and operational tools have been adopted by electricity value chain stakeholders (Transmission System Operators (TSOs), Distribution System Operators (DSOs), RES plant operators, aggregators and forecast service providers) in applications including power system scheduling, the estimation of reserves, congestion management, the coordination of renewables with storage or trading in electricity.
Originating from meteorology, and not initially oriented to the energy sector, numerical weather prediction (NWP) tools have been developed. The NWP models are a result of collaborations with energy and meteorology, with the aim of aligning NWP models to the expectations of the power and energy industry. These models are used to forecast wind, together with algorithms that provide the non-linear transformation of wind speed into power considering the other relevant meteorological and orographic effects, in addition to the wind turbine type and / or wind farm layout, including shadow effects.
The prediction type of wind speed and power has different definitions according to the observation cycle duration: ultra-short-term, short-term, medium-term and long-term predictions [1]. Depending on the forecast time horizon, such forecasts are used by grid operators for intraday and near-to-real time grid operations (few hours ahead, 15 to 30 min), day-ahead market clearing check (24 hours ahead) and operational planning (hundred hours ahead).
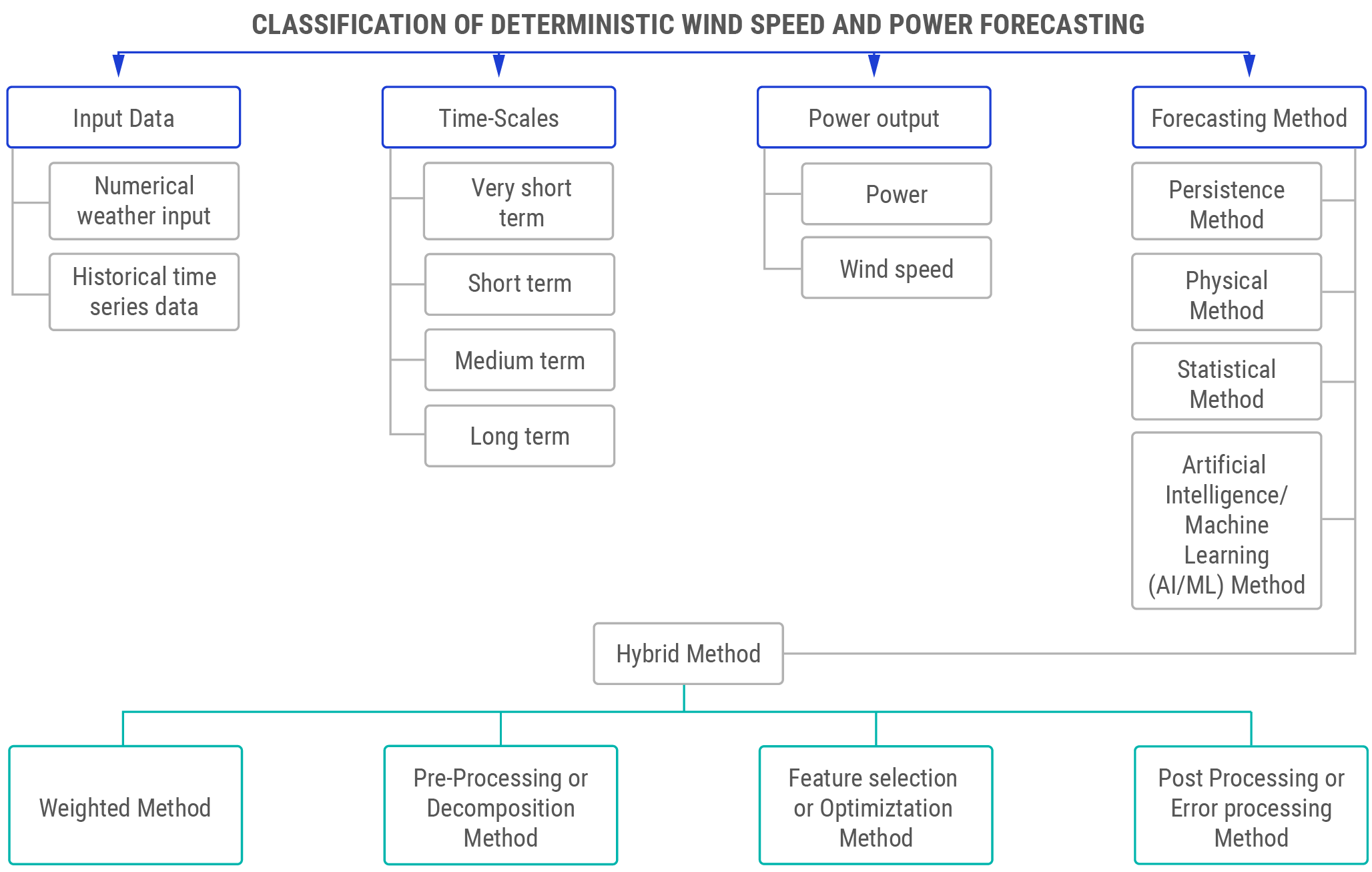
RES forecasting involves a model and value chain encompassing models for weather forecasting and for RES forecasting as well as models related to the applications the forecasts are used for (i.e. decision making for trading). A detailed classification of deterministic wind speed and power forecasting approaches is shown in the figure above. Forecasting approaches can be split into:
- Persistence approach: this approach was used in the past to be compared with novel short-term forecasting methods to identify their improvements.
- Physical approaches: physical methods use detailed physical models of wind turbines/farms. The modelling effort requires a description of the area, such as roughness and obstacles as well as weather forecasting data of temperature, pressure, etc. Although it is appropriate for medium to long-term wind power prediction, it is computationally complex and therefore requires considerable computing resources.
Statistical-based approaches: these approaches use historical time series and are split into:
- Time series-based: the moving average model (MA), the autoregressive model (AR) or a combination called the Auto-Regressive Moving Average model (ARMA). These models are suited to short-term wind power forecasting.
- Artificial Neural Network-based (ANN): ANNs can identify non-linear relationships between input features and output data.
- Hybrid approaches: these approaches combine two or more models of similar or different nature. In statistics and machine learning, diverse predictive models are developed by using multiple algorithms and different training datasets.
Benefits
Enhanced wind forecasting will:
- Avoid trading energy as a bulk and match demand thanks to wind forecast at the minute level;
- Reduce the need for energy balancing from the reserve markets, which are more costly than letting the energy market balance the system;
- Improve the flexibility and possibility for wind to participate in the reserve markets; and
- Ensure the robust optimisation of the grid integration issues of wind power and distribution networks using AI techniques.
Current Enablers
There are two critical enablers to reach wind forecasting results:
- The input data from sensors external to the modelling tool developers, which can be used fruitfully by the downstream modelling teams to predict wind in-feeds.
In ref [2], new meteorological features to improve the accuracy of wind power forecasts are investigated, using the Principal Component Analysis (PCA). A wrapper feature selection approach is adopted by using NWP data that are publicly available. The meteorological feature selection is performed using the ANN approach. The proposed methodology is then applied to seven wind parks located in Portugal which exhibit distinctive climatic conditions. This enables the identification of similarities in the NWP meteorological features that contribute to improving the forecast. At the same time, they enable the performance of the forecast to be compared among the wind parks available (see below).
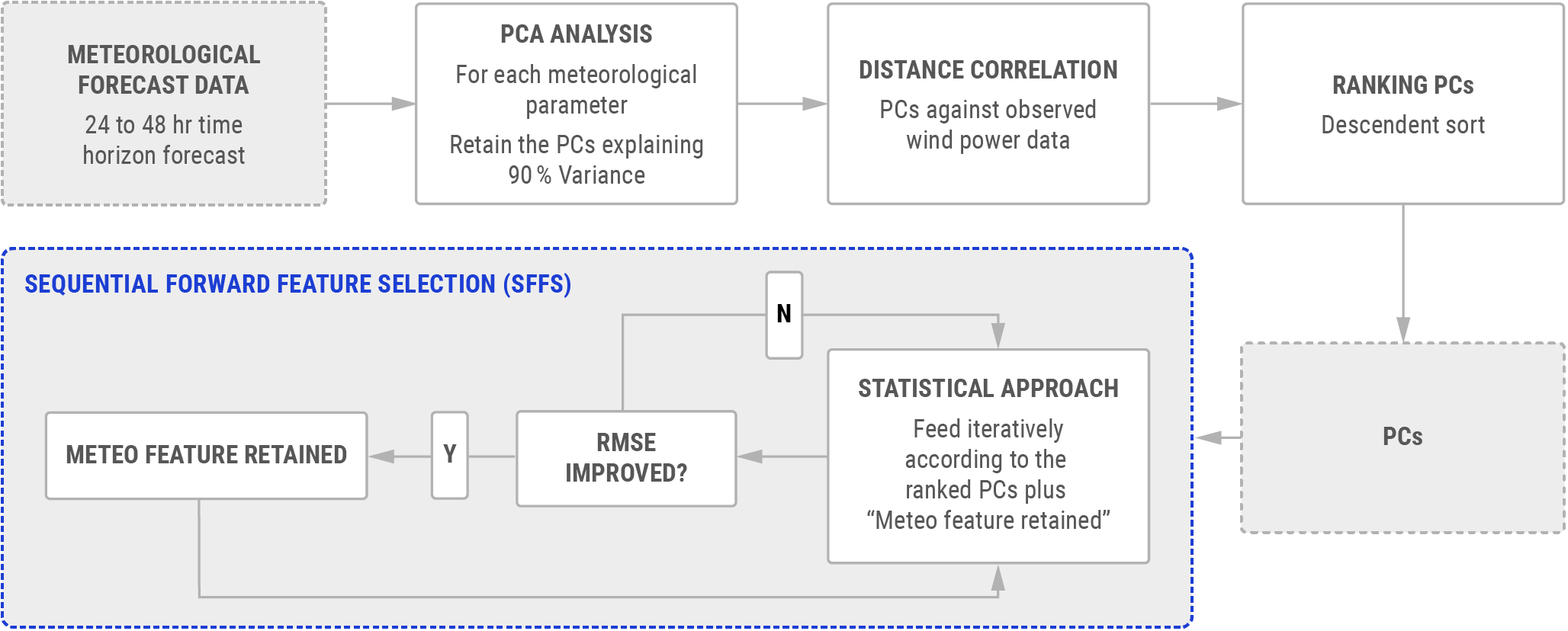
This paper concludes that the use of key meteorological parameters such as wind gusts, wind power density, wind shear and planetary boundary layer height can be used to improve the forecast of wind power. This methodology leads to an improvement in the forecast performance, ranging between 13% (wind park 1) and 37% (wind park 4) in the case of the normalised root mean square error (RMSE). The authors claim that future work should focus on understanding the impact of using meteorological features that explain a reduced variance as well as the use of other objective functions in the feature selection algorithm (e.g. the maximisation of the remuneration in the wholesale electricity market).
Reference [1], published in 2023, extensively covers the literature dedicated to the recent wind power generation forecasting technologies (151 papers since 2020). Most models:
- Use NWP and on-site measurement data (SCADA) as the basis;
- Read the data from monitoring points; and
- Then use the obtained wind speed and output data to predict wind power.
However, due to the confidentiality of data sources, most prediction models rarely have a complete theoretical basis and historical data. Hence, the research is limited to a single site and region. For multiple regions or large-scale spatial prediction at scattered meteorological stations, the results can still be questioned.
- The software tools and techniques which attempt continuous improvement of the forecast performance.
Reference [1] lists 15 operational tools where some wind power generation forecasting systems are currently used. They encompass wind speed prediction, wind power modelling and wind power forecasting for single turbines and wind farms.
R&D Needs
The R&D topics of major importance for improving on power forecasting performance [1, 3] are listed below:
- Meteorological variables prediction: accounting for the influence of the physical environment by incorporating different time horizons of future meteorological data as an input factor.
- Wind turbine modelling: accounting for the influence of the physical wind turbine characteristics.
- Wind field modelling: more suitable identification functions to account for different wind fields, together with artificial intelligence methods such as neural networks or deep learning methods.
- Short-term wind power forecasting of wind farms: accounting for short-term wind power forecasting (induced by wind speed drops) under the actual operation scenarios of wind farms.
- Error transfer mechanisms from wind speed forecasting to wind power forecasting: accuracy of short-term wind power forecasting is enhanced by correcting NWP data.
- Accuracy and speed of prediction of big data: use parallel modelling of the prediction algorithm, incorporate spatial correlation features into the classification and prediction, and verify the feasibility of the model by using different datasets with the development of new or the integration of multiple optimisation algorithms.
- Sufficient data management: to ensure handling of dependability of several interconnected forecasting models and handling data privacy, and to harmonise data structures by using standard data processing protocols.
The quality of wind forecasting techniques must inexorably improve, which will impact the efficiency of the whole electricity system. Such improvements will come from the combination of several intertwined levers, as demonstrated in the project SMART4RES [3, 4], funded by the European Commission:
- Forecasting techniques using new fine-tuned and adapted numerical models;
- Improving the quality of the input data;
- Using computational and data storage facilities, the performance of which is continuously evolving; and
- Using innovative decision-making tools which involve such forecasts in end-use applications.
The technology is in line with milestone “Integration of dynamic ratings and AI-based renewable power forecasts” under Mission 1 of the ENTSO-E RDI Roadmap 2024-2034.
TSO Applications
In Ref [1], the comparison of 106 published approaches is proposed using a two criteria analysis: the forecasting accuracy and the time resolution.
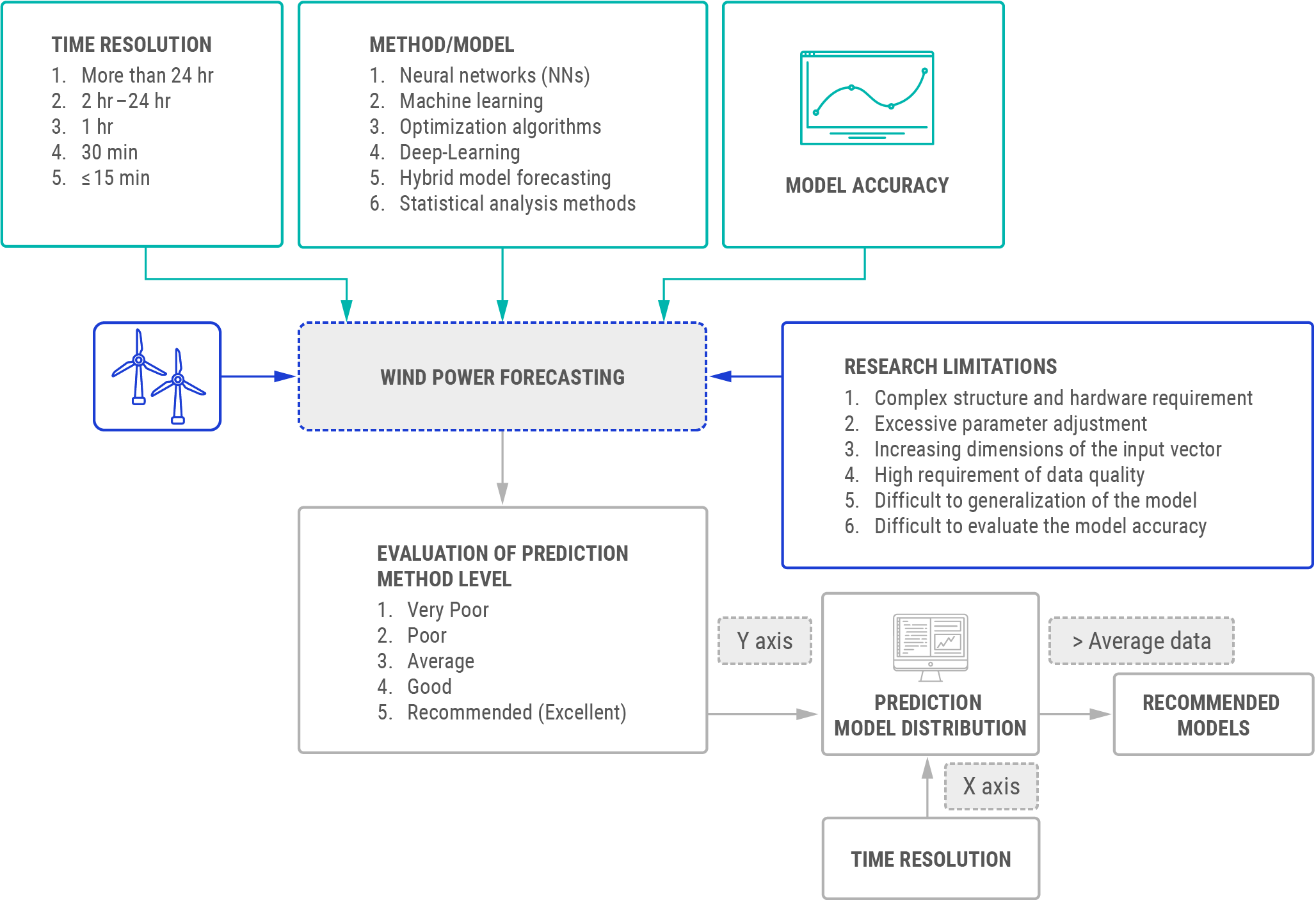
The most promising tools developed and published so far at research level are referenced in Ref [5, 6, 7, 8] according to the review done in [1].
Examples
Location: Europe [3, 4] | Year: 2023 (project started in 2019) |
---|---|
Description: The Smart4RES project aimed at the research, development and validation of the next generation of tools for modelling and forecasting energy production from variable renewables and decision aid for a number of use cases. | |
Design: Smart4RES proposed disruptive research ideas for developing and validating the next generation tools, ensuring an increase in RES forecasting performance. The increased forecasting performance had a leverage on the economic value of RES by considering the whole value chain, from weather forecasting to end-use applications. | |
Results: The project achieved a 15% improvement in RES forecasting performance. New tools have been developed to enhance short-term forecasts and high resolution weather predictions. Moreover, the project tackled data privacy issues to allow for data sharing between RES plants to improve forecasting performance. Lastly, the project developed analytics tools which combine forecasting and optimisation to improve grid management-related decisions. |
Location: Germany [9] | Year: 2021 (project started in 2018) |
---|---|
Description: The ParkCast project addressed the optimisation of minute-scale power forecasts of offshore wind farms using long-range lidar measurements and data assimilation. It aimed to develop, optimise and evaluate new methods for short-term forecasts of the performance of offshore wind farms. The power forecasts focused on the time range up to 60 minutes with high temporal resolution. The aim was to significantly improve the temporal resolution and forecasting quality of the parking performance in the above-mentioned time period and thus contribute to grid stability and security of supply. | |
Design: To this end, long-range lidar measurement data were assimilated into a high-resolution, local weather model using new methods based on machine learning. Physical and advanced machine learning-based prediction models were then used for the power prediction and validated in real time for the Alpha Ventus Offshore Wind Farm as part of an online test phase. | |
Results: ParkCast contributed to forecast accuracy and grid operations stability due to the enhancements of short-term wind offshore power forecasting. |
Location: Norway [10] | Year: 2018 |
---|---|
Description: A comparative study assessing the potential benefit of using a deterministic NWP model with 1 hour generation time compared to an NWP ensemble with 2.5 hours generation time. | |
Design: Nine months of data for the Norwegian wind farms Bessakerfjellet and Hitra were organised to evaluate several forecast models, based on various uses of the predictive information. Hourly quantile wind power forecasts were made. | |
Results: In terms of mean absolute errors, the results were neutral. However, in situations where moderate to large changes in wind speed were forecast, the scores were in favour of the deterministic NWP model with 1 hour generation time. |
Technology Readiness Level The TRL has been assigned to reflect the European state of the art for TSOs, following the guidelines available here.
- TRL 9 for the 15 operational tools listed above in the current enablers.
- TRL 4 to 7 for the most promising tools listed in the TSO applications.
References and further reading
T. Wen-Chang et al., “A Review of Modern Wind Power Generation Forecasting Technologies,” Sustainability, vol. 15, no. 14, p. 10757, July 2023.
L. Andrés et al., “Wind power forecasting using ensemble learning for day-ahead energy trading,” Renewable Energy, vol. 191, pp. 685–698, May 2022.
European Commission. “Next generation modelling and forecasting of variable renewable generation for large-scale integration in energy systems and markets.”
A. Lagos et al., “State-of-the-Art using bibliometric analysis of wind-speed and power forecasting methods applied in power systems,” Energies 2022, vol. 15, no. 18, p. 6545, Sep. 2022.
G. An et al., “Short-Term wind power prediction based on particle swarm optimization-extreme learning machine model combined with adaboost algorithm,” IEEE Access 2021, vol. 9, pp. 94040–94052.
Z. Sun and M. Zhao, “Short-term wind power forecasting based on VMD decomposition, convLSTM networks and error analysis,” IEEE Access 2020, vol. 8, pp. 134422–134434, 22 Jul. 2020.
W. Dong et al., “Short-term regional wind power forecasting for small datasets with input data correction, hybrid neural network, and error analysis,” Energy Rep. 2021, vol. 7, pp. 7675–7692, Nov. 2021.
Rave Research at Alpha Ventus. “Parkcast.”
J. B. Bremnes, “On the use of NWP forecasts in wind power forecasts for the next few hours” Met Report, May 31, 2018.